Imagine you could suddenly get 20 times more mileage from your car on a full tank or electric charge. That would be a game-changer. Now imagine an overnight improvement of 95% in a business or industry’s efficiency.
This is the calculus global markets had to contend with in January 2025 when Chinese artificial intelligence (AI) firm DeepSeek hit the scene. The previously little-known start-up set off panic with the release of its AI app-based chatbot, DeepSeek-R1. Why, and what does it mean for the development of AI and the companies spending hundreds of billions of dollars in the AI arms race?
What did markets make of this upstart?
DeepSeek-R1 was launched with the claim that its performance is on par with some of the most advanced models being developed by AI leaders such as Google, OpenAI, and Meta – and that it can achieve this with a 95% improvement in training efficiency. In other words, the open-source chatbot was created at a fraction of the cost of other leading language learning models.
The markets’ approach was to shoot now and ask questions later. Investors took this development as evidence that the AI revolution won’t need anywhere near the high level of computing power currently priced into shares. That impacted chipmaker Nvidia most drastically. The American firm, best known for designing ultra-potent semiconductors that power most high-end AI technology, faced an unprecedented selloff. Over half a trillion dollars was wiped from its share capital in one day of trading.
What is DeepSeek-R1?
DeepSeek-R1 is an AI model developed by Chinese artificial intelligence start-up DeepSeek. R1 holds its own against (and in some cases surpasses) the reasoning capabilities of some of the world’s most advanced foundation models — but at a fraction of the operating cost, according to the company. R1 is also open-sourced under an MIT license, allowing free commercial and academic use.
Source: Builtin.com
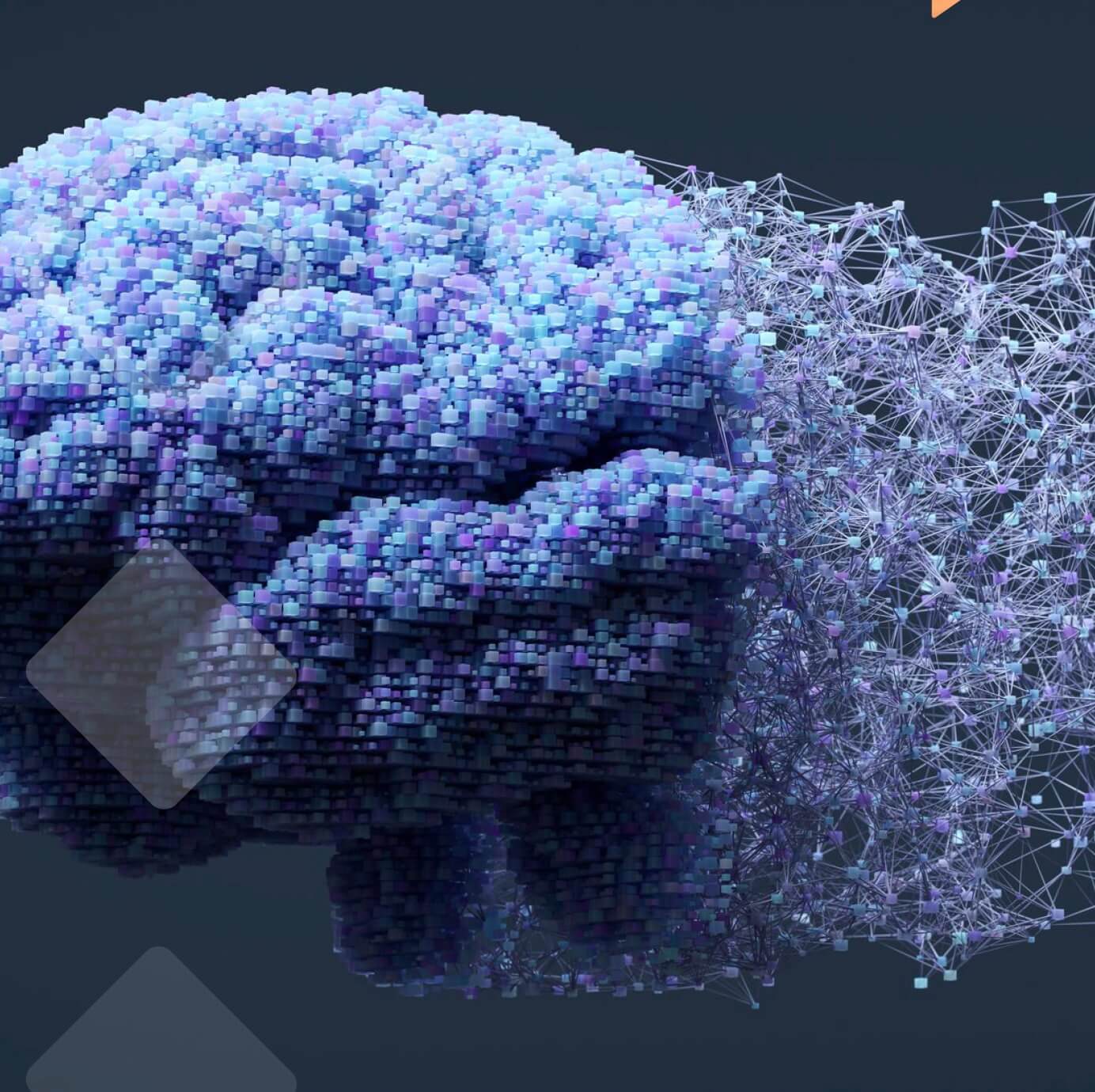
How did they do that?
Insiders have delved into DeepSeek’s efficiency claims. While the technical details are complex, the core principle involves optimising how the model processes and learns from data. Imagine a teacher training a student: instead of overwhelming them with information, the student is given carefully curated examples and allowed focused practice, leading to faster, more effective learning. DeepSeek-R1 likely employs similar techniques, streamlining the learning process and reducing the computational resources required.
This mixture of experts (MoE) methodology essentially uses many smaller models (called “experts”) that are only active when required so that performance is optimised and computational costs are reduced. Although DeepSeek has not confirmed it, it is widely believed that its R1 model relied heavily on other AI models. In this case, OpenAI’s GPT-3 model was the experienced teacher (a large, powerful “teacher” AI model) used to impart its knowledge to a younger, less experienced student (a smaller, more efficient “student” model). Having been trained on a massive dataset, the teacher model distils its essential insights into a more compact form that the student model can readily absorb.
And this is where the devil comes in the details. Without having to bear the cost of developing the teacher model, the true cost of building a better model, like DeepSeek-R1, remains unknown.
Was the Nvidia sellout an overreaction?
If nothing else, the Nvidia selloff may have been poorly informed. This is often the case when panic sets in.
It appears that the sellers’ thinking was that “since we can now do AI training with vastly superior efficiency, less computing power would be demanded”. That reasoning holds in a strict sense. Yes, if DeepSeek’s 95% claim holds, we can build an AI model with 5% of the computing power we previously believed to be required.
However, even if the claim holds up, a paradox of human behaviour must not be ignored. The Jevons paradox suggests that improvements in the production of a resource lead to increased consumption. The classic example is the increased use of coal during the Industrial Revolution in Britain, thanks to new ways of burning it more efficiently. As the amount of coal required to produce a unit of energy decreased, the demand for coal grew even more as new uses were developed and industrial technologies became widespread throughout the economy.
In more recent times, the falling cost of mobile data has unleashed an insatiable demand for it. Now that we can effortlessly share high-resolution videos and stream endless hours of YouTube on our phones, mobile data traffic has grown exponentially, more than doubling every two years.
Applied in the AI context, the Jevons paradox means that more efficient models won’t necessarily dampen demand for computational muscle. Instead, they could enable the development of even larger and more complex AI systems, ultimately driving up demand for computing power.
Greater efficiency almost always leads to greater demand
Even without the dramatic efficiency gains promised by DeepSeek-R1, the demand for more advanced AI means that the overall market for Nvidia’s hardware will likely continue to grow. Greater efficiency can also boost demand by making more and more use cases viable. Many of these are on the horizon. One worth mentioning is the pursuit of agentic AI. This refers to AI systems that can independently plan and execute complex tasks. Imagine an AI assistant that not only answers questions, but also proactively manages your schedule, handles your banking, and even anticipates your needs. Agentic AI will no doubt be widely adopted, but it will require an enormous leap in computing power and algorithmic sophistication to get there, further fuelling the demand for advanced hardware and software. Seen as the holy grail, the cloud hyperscalers – Amazon Web Services, Microsoft, Google, and Meta – are pouring hundreds of billions of dollars into building the AI infrastructure, training the models, and developing the applications agentic AI needs to succeed.
How should investors interpret the battle of the AIs?
All of this suggests a reversion to the mean as markets selfcorrect. Indeed, Nvidia’s share price has begun to recover from January’s selloff. As Amazon’s Chief Executive, Andy Jassy, recently pointed out, most AI computing is driven by Nvidia chips, and Amazon has “a deep partnership with Nvidia and will for as long as we can see into the future”.
While the AI landscape is evolving rapidly, we remain confident in the long-term fundamentals of Nvidia and other hyperscalers. In particular, Nvidia’s dominance in developing graphic processing units and the hyperscalers leadership in building out cloud computing at scale, coupled with the insatiable demand for AI, positions them as key beneficiaries of this transformative technology.